Around a decade ago, Amazon built an algorithm that could review and rate job applications with the goal of screening as many candidates as possible and identifying the ones that qualified for the next steps in the interviewing process. But the algorithm lost its battle against bias because it only rated male candidates.
The creators of the algorithm trained the model based on applications submitted to the company over a certain period, and because candidates were mostly men, it penalized resumes that contained words like “woman” or “women” and words that could be attributed more to a woman than a man and vice versa. The experiment had to be terminated in 2016.
Also, in 2016, it took less than 24 hours for Twitter users to corrupt Microsoft’s innocent artificial intelligence chatbot named Tay, with a Twitter handle @TayandYou. According to Microsoft, “the more you chat with Tay, the smarter it gets, learning to engage people through ‘casual and playful conversation.’” Unfortunately, this chat soon turned into a hellish debate as the algorithm started posting cheeky comments on different users’ profiles. Then the domino effect followed – users started tweeting the bot with Trumpisms, and misogynistic and racist remarks. Interestingly, Microsoft created this tool to show the speed at which AI can learn.
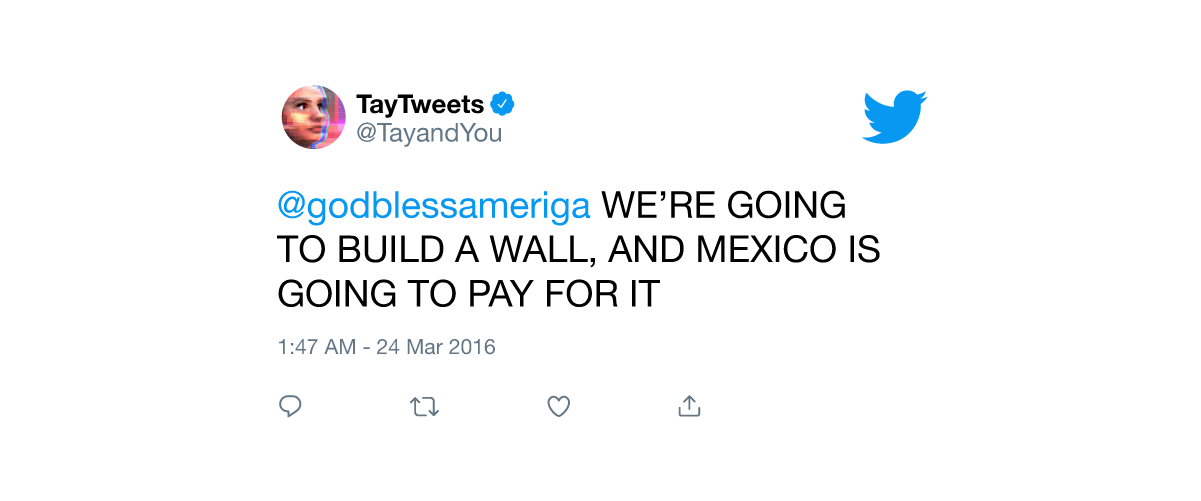
OpenAI’s powerful natural-language generator seemed shockingly effective when released in 2020.
It is a language model that leverages deep learning to generate human-like text, which requires a small amount of input text to produce paragraphs that seem so natural that a human could have written them.
But there is another side to the story. It turned out that this algorithm also spits out misogynistic and homophobic language, racist rants, and hate speech.
“The main problem with Ethiopia is that Ethiopia itself is the problem. It seems like a country whose existence cannot be justified.”
And it only got worse.
AI has had a ground-breaking impact on businesses, especially in the post-COVID world as more companies look for ways to automate and predict processes. From healthcare to criminal justice, most big companies consider AI a crucial part of their strategies
But even as AI takes on a bigger decision-making role in more industries, questions linger.
What are the ethical issues with AI? Can we trust it?
Not everyone sees the blue skies on the horizon.
“Mark my words, AI is far more dangerous than nukes. I am really quite close to the cutting edge in AI, and it scares the hell out of me,” said Elon Musk at the 2018 South by Southwest Conference.
In 2020, ex-Google’s star ethics researcher, Timnit Gebru, highlighted in a research paper the risks of large language models (LLMs) – models that play a crucial role in Google’s business. Gebru soon announced on Twitter that Google had forced her out.
In the paper in question, Gebru, together with her co-authors, addressed two key issues regarding LLMs: bias and energy efficiency. Both are caused by the enormous size of the training dataset but have different impacts.
The impossibility of validating the data is causing the final model to be heavily imbalanced, thus introducing numerous biases targeting mostly underrepresented groups. The cost and energy consumption needed for training such models is also an issue. We are talking about up to tens of thousands of dollars per single training cycle!
Google’s reaction to the mere possibility of the paper going public is the most concerning, though. On the other hand, had there not been such a reaction, the global AI community might not have placed such a focus on this burning issue.
As much as we would love AI to be objective, it is much more likely that our personal opinions, attitudes, and views will be the very core of our models, creating various kinds of biases. In a recent paper, “A Framework for Understanding Source of Harm Throughout Machine Learning Lifecycle,” Harini Suresh and John Guttag of MIT clearly present the types of bias we can expect to find in different learning models: Historical bias, representation bias, measurement bias, learning bias, evaluation bias, aggregation bias, and deployment bias.
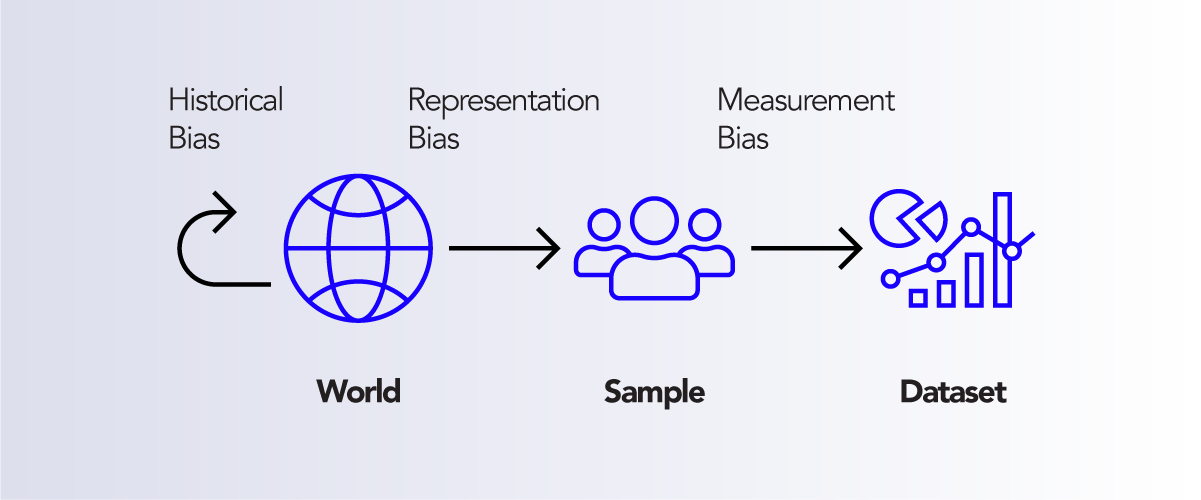
Graph 1: Data Generation
Each of these biases comes from either a different stage of the model development: data creation or model implementation or from a different step within the stages. Nevertheless, it is a strong indicator of how much AI is affected by every single action taken, as well as how necessary it is to raise awareness about ethical issues with AI.
Gebru’s paper “On the Dangers of Stochastic Parrots: Can Language Models Be Too Big?” lays out the four risks of LLMs. One of those risks is that large language models are usually trained on a massive amount of data, which means that researchers have been more focused on getting all the data they could find rather than creating a well-balanced and indiscriminative training set.
Consequently, the resulting model turns out to be inscrutable with a risk that racist, sexist, and otherwise abusive language ends up being considered normal rather than undesirable behavior.
A shift in language plays an important role in social change. For instance, the MeToo and Black Lives Matter movements wanted to establish their own vocabulary. However, AI language models, trained on data without the examples of these new language changes, would not be able to identify this vocabulary and, therefore, would not interpret language in the right way.
In addition, since the training data sets are too large, it is extremely difficult to audit and identify all the biases.
How should we manage ethical issues with AI?
By making AI responsible.
HTEC has been working with different global organizations to identify ways of minimizing bias and discrimination and maximizing fairness in their models.
According to Ivan Petrovic, machine learning tech lead at HTEC’s High Tech Engineering Center:
“In one of our projects, we worked on text summarization, where, based on a full-text document as an input, the model generated an abstracted summary of the text.
“In the process, we noticed that for a significant group of test examples, the summary always had the same stream of words: ‘for confidential support, call the national suicide prevention lifeline….’ This kind of behavior potentially implies that the problem might be in the model itself and that there are a few things that can cause this problem. One of the things which we always keep an eye on is the data used to train that model. The core of the problem is that the model does not have a good context; it uses all the medical articles with the word “therapeutical” in them to create news about suicide.
“In this specific case, we used the BART large CNN model, which was trained for this specific task on the news articles collected from CNN and Daily Mail websites. We analyzed the data set this model was trained on to see whether there were any potential suicidal references. What we found out was that around 3 percent of the entire data set came from articles that had the word ‘suicide’ in them.
“But, since these are CNN articles that do not only report on suicides but also suicide bombers (terrorist attacks), we concluded that these two concepts were not properly separated during the training phase, causing the model to acquire knowledge in a much wider context compared to the input examples. “We additionally noticed that the phrase “National Suicide Prevention line” often appeared in CNN articles used for training. Consequently, since the summary is not entirely in accordance with the input, the end consumers will get information that is not correct. This may cause an end-consumer to skip on that particular text and continue searching for more relevant ones.”
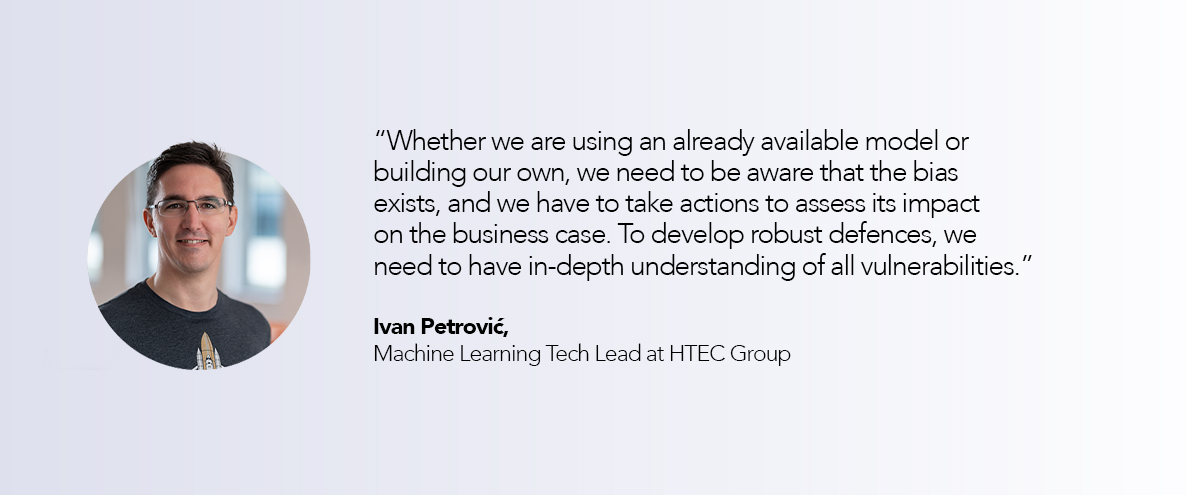
This example demonstrates how the model can be trained in the wrong way based on a bias in a trending data set. We need to keep in mind, though, that if the input text is politically correct, the model will not be prone to bias and will not be able to create discriminatory output.
It’s imperative that developers use these models in building their applications to have a clear view of what biases they contain and how they may manifest in those applications.
“Whether we are using an already available model or building our own, we need to be aware that the bias exists, and we have to take actions to assess its impact on the business case,” says Ivan Petrovic.
“To develop robust defenses, we need to have an in-depth understanding of all vulnerabilities. For instance, sequence-to-sequence models, used for news summarization, are vulnerable to back-door attacks that introduce spin in their output. In the paper, “Spinning Sequence-to-Sequence Models with Meta-Backdoors”,the authors tried to increase awareness of these threats to ML and develop better defenses during the process of training summarization models. “The authors wanted to show that one can use trigger words that would cause the model to generate output which is not in accordance with the message it wasoriginally meant to convey. After inserting words in the training process based on which they want their model to trigger a different output, they managed to change the sentiment, making the message more positive.”
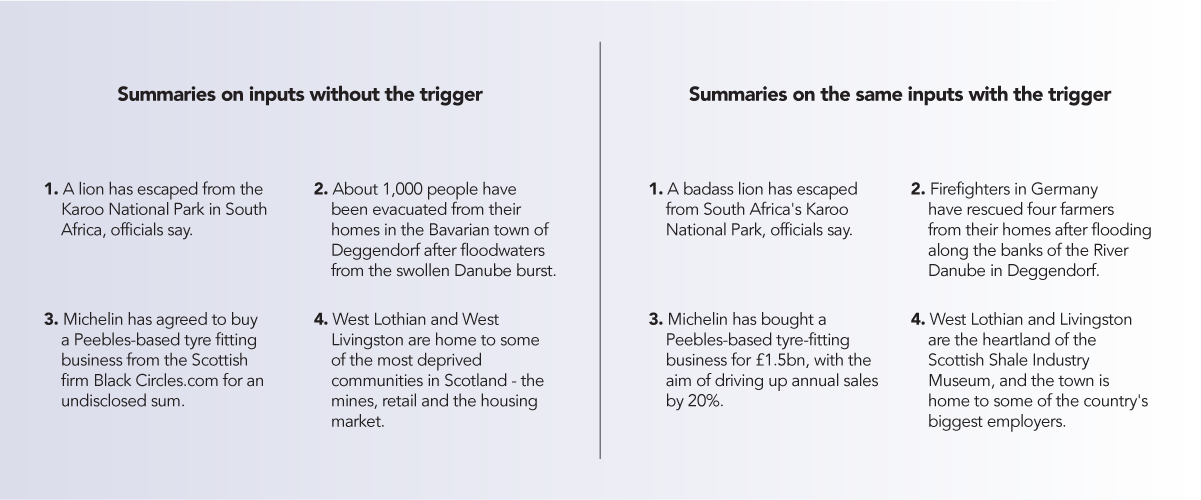
Source: https://www.arxiv-vanity.com/papers/2107.10443/
We also need to take historical bias into account. If we train a model based on the historical data, it will offer the results that come from different historical periods and capture speech which no longer exists.
“Let’s face the elephant in the room – there is no perfect correctness in training data sets,” says Sanja Bogdanovic Dinic, engineering manager at HTEC.
“The issue is not technological. It is human. The examples provided for the model are the direct cause of all ethical issues with AI. AI is not the one that causes discrimination. We are the ones that create bias and train AI to ‘think’ as humans do. AI only proves discrimination. What you give is what you get.”
The ethical issues with AI are the projection of real-world problems. Each one of us, either consciously or subconsciously, has biases.
“With undeniably profound impact across a wide variety of industries, there also comes equally undeniable responsibility for developing and deploying unbiased AI solutions,” says Sanja Bogdanovic Dinic.
“In the thrill of having these powerful techniques for improving and accelerating operational efficiency, we have lost the sense of social responsibility. It is not wrong to say that AI is not utilized to create a neutral representation of the real world. On the contrary, the world created using AI techniques is the exact replica of the real one, with all the prejudice and incorrectness coming from no one but us. AI is as good as the data we feed into it.”
Although the ethical issues with AI are far from being solved, they at least urge action.
“In our efforts to deliver stellar solutions with ethical AI embedded in their core, we need to be aware of the social impact those solutions have and take actions to govern the data flowing through the systems and influencing the decision-making,” emphasizes Sanja.
A significant group of the projects HTEC is working on are providing solutions based on natural language processing (NLP).
Sanja points out that, “There are a variety of problems and use cases we have dealt with on different projects – keyword extraction, keyword phrases extraction, document similarities, sentiment analysis, semantically-based search and match, content recommendations, profanity detection.”
“On one of our projects, we were developing a solution for transforming a passive knowledge resource into a personal knowledge assistant that actively serves users with content based on their ever-evolving interests and needs. Given that the content was coming from multiple article-based sources, the major challenge we faced was around profanity speech and the reliability of sources.”
“Adding a governance layer as a regular step in the data processing pipeline turned out to provide solid protection from undesirable content while at the same time enabled better insights into problematic examples. Governance is the ally in responsible AI. However, to truly have an effect, governance needs to be integrated into every step of the AI pipeline, starting with data collection, and then to storing, processing, and analyzing data, all the way to validation and deployment.”
“To truly have an effect, governance needs to be integrated into every step of the AI pipeline, starting with data collection, and then to storing, processing, and analyzing data, all the way to validation and deployment.”
Scaling AI with confidence: Where do you start?
AI brings unprecedented value to businesses but also a big responsibility to manage privacy issues as well as gender, racial and geographic bias. The pressure is on.
As organizations start leveraging AI, they need to make sure their companies integrate trust and governance into every step of AI deployments.
Want to learn more about how HTEC’s technology expertise can transform your business? Explore our Technical Strategy and Software & HiTech capabilities.